Modern bioprocess management, guided by sophisticated analytical techniques, digitalization, and automation, is generating abundant experimental data crucial for process optimization. Machine Learning (ML) techniques have proven crucial in analyzing these huge datasets, allowing for the efficient exploration of design spaces in bioprocessing. ML techniques are utilized in strain engineering, bioprocess optimization, scale-up, and real-time monitoring…
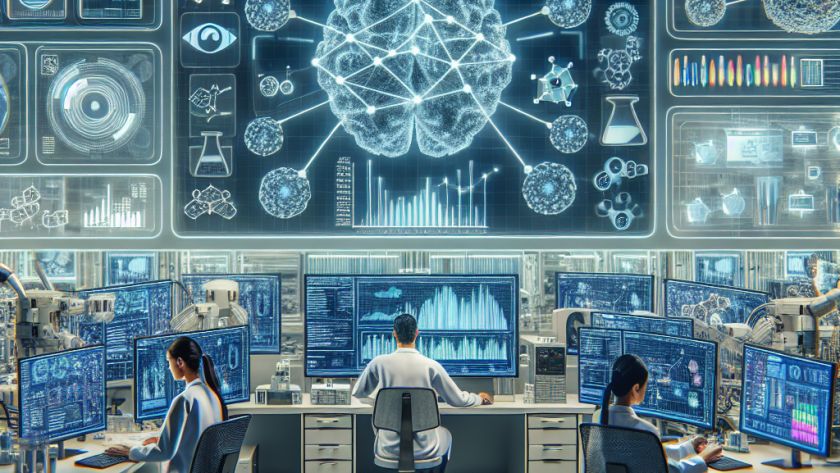