The human face serves an integral role in communication, a feature that is not lost on the field of Artificial Intelligence (AI). As AI technology advances, it is now creating talking faces that mimic human emotions and expressions. Particularly useful in the area of communication, the technology offers numerous benefits, including enhanced digital communication, higher…
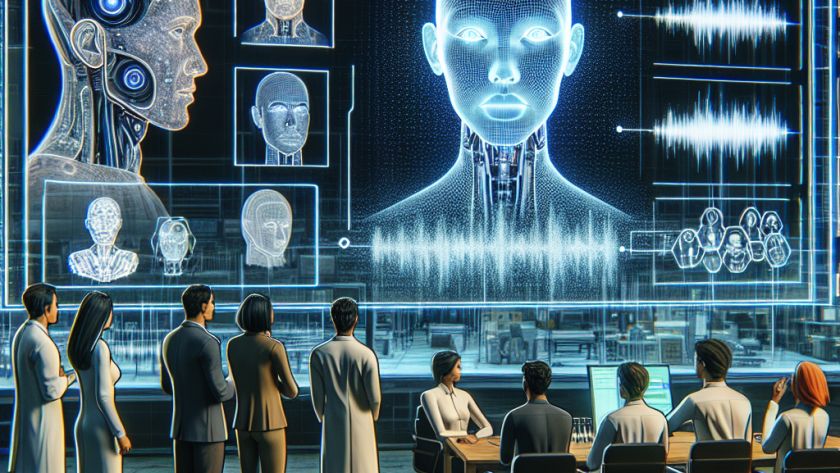