


As AI systems continue to advance, researchers and policymakers are concerned about ensuring their safe and ethical use. The main issues center around the potential risks posed by ever-evolving and increasingly powerful AI systems. These risks involve potential misuse, ethical issues, and unexpected consequences stemming from AI's expanding abilities. Several strategies are being explored by…
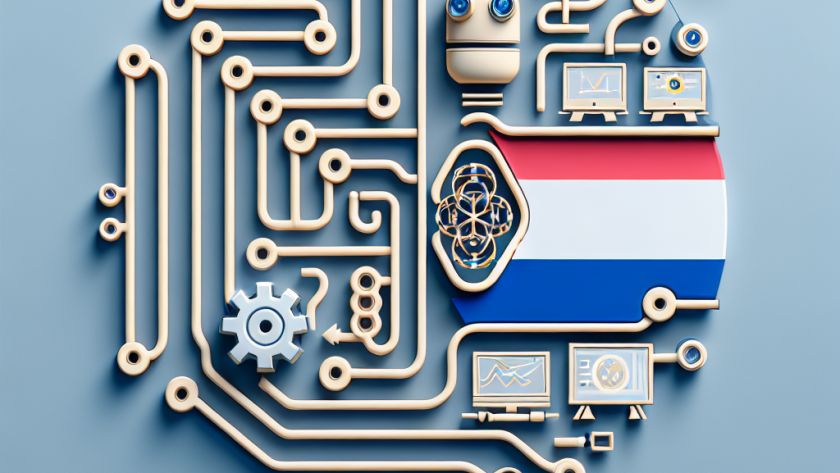
Automated Machine Learning (AutoML) has become crucial for data-driven decision-making, enabling experts to utilize machine learning without needing extensive statistical knowledge. However, a key challenge faced by current AutoML systems is the efficient and correct handling of multimodal data, which can consume significant resources.
Addressing this issue, scientists from the Eindhoven University of Technology have put…

The existing Artificial Intelligence (AI) task management methods, including AutoGPT, BabyAGI, and LangChain, often rely on free-text outputs, which can be lengthy and inefficient. These frameworks commonly struggle with keeping context and managing the extensive action space linked with arbitrary tasks. This report focuses on the inefficiencies of these current agentic frameworks, particularly in handling…




Large language models (LLMs) have gained significant popularity recently, but evaluating them can be quite challenging, particularly for highly specialised client tasks requiring domain-specific knowledge. Therefore, Amazon researchers have developed a new evaluation approach for Retrieval-Augmented Generation (RAG) systems, focusing on such systems' factual accuracy, defined as their ability to retrieve and apply correct information…

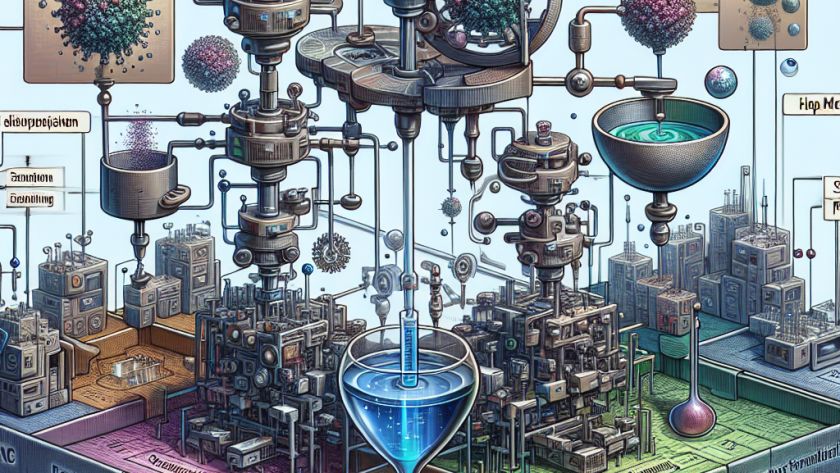
Reinforcement Learning from Human Feedback (RLHF) plays a pivotal role in ensuring the quality and safety of Large Language Models (LLMs), such as Gemini and GPT-4. However, RLHF poses significant challenges, including the risk of forgetting pre-trained knowledge and reward hacking. Existing practices to improve text quality involve choosing the best output from N-generated possibilities,…


Large Language Models (LLMs) have improved significantly, but challenges persist, particularly in the prefilling stage. This is because the cost of computing attention increases with the number of tokens in the prompts, leading to a slow time-to-first-token (TTFT). As such, optimizing TTFT is crucial for efficient LLM inference.
Various methods have been proposed to improve…