


The accuracy of Large Language Models (LLMs) such as Google's GPT (Generative Pre-trained Transformer) is vital, particularly when it comes to producing content that needs to be factually correct, such as educational content or news reports. However, despite their abilities, LLMs often generate plausible but incorrect information, a phenomenon known as "hallucination."
Google AI researchers have…


Community Question Answering (CQA) platforms like Quora, Yahoo! Answers, and StackOverflow are popular online forums for information exchange. However, due to the variable quality of responses, users often struggle to sift through myriad answers to find pertinent information. Traditional methods of answer selection in these platforms include content/user modeling and adaptive support. Still, there's room…

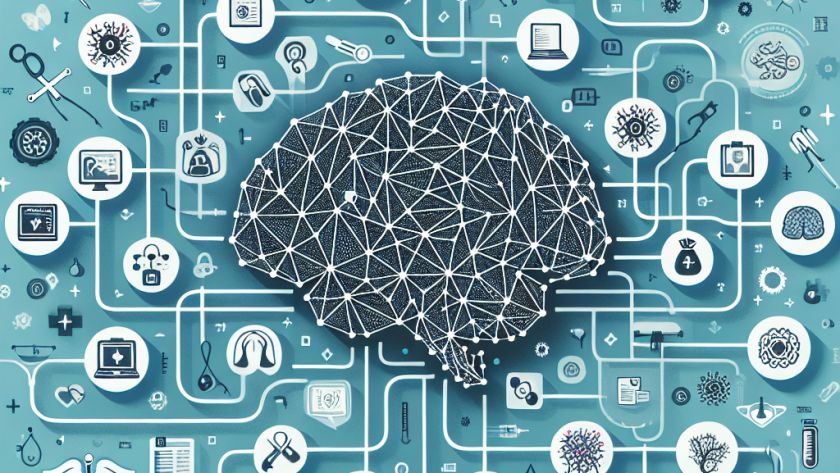
Biomedical data is increasingly complex, spanning various sources such as electronic health records (EHRs), imaging, omics data, sensors, and text. Traditional data mining and statistical methods struggle to extract meaningful insights from this high-dimensional, heterogeneous data. Recent advancements in deep learning offer a transformative solution, enabling models that can directly process raw biomedical data. Such…
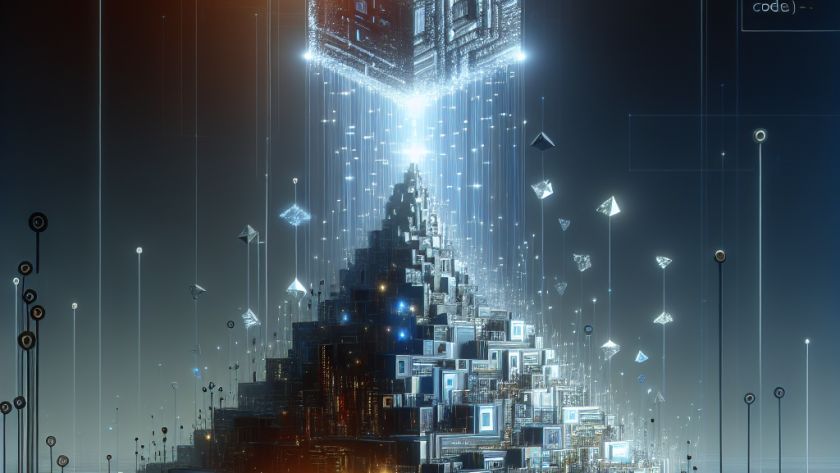
Artificial Intelligence (AI) and data science are fast-growing fields, with the development of Agentic Retrieval-Augmented Generation (RAG), a promising evolution that seeks to improve how information is utilized and managed compared to current RAG systems.
Retrieval-augmented generation (RAG) refines large language model (LLM) applications through the use of bespoke data. By consulting external authoritative knowledge bases…

Research conducted by DeepMind has shed new light on the complexities of machine learning and neural representation, providing insights into the dissociations between representation and computation in deep networks. High capacity deep networks frequently demonstrate an implicit bias towards simplicity amidst their learning dynamics and structure. The employed simple functions allow for easier learning of…

The InternLM research team is dedicated to improving and developing large language models (LLMs) specifically tailored for mathematical reasoning and problem-solving. They aim to strengthen artificial intelligence's performance ability when dealing with mathematically complex tasks, such as formal proofs and informal problem-solving.
Researchers from several esteemed institutions have worked together on producing the InternLM2-Math-Plus model…


Artificial Intelligence (AI) has witnessed significant breakthroughs in image generation in recent years with four models, DALL-E, CLIP, VQ-VAE-2, and ImageGPT, emerging as game-changers in this space.
DALL-E, a variant of the GPT-3 model, is designed to generate images from textual descriptions. Taking its name from surrealist Salvador Dalí and Pixar’s WALL-E, DALL-E boasts creative skills…

An AI's understanding and reproduction of the natural world are based on its 'world model' (WM), a simplified representation of the environment. This model includes objects, scenarios, agents, physical laws, temporal and spatial information, and dynamic interactions, allowing the AI to anticipate reactions to certain actions. The versatility of a world model lends itself extremely…