Large Language Models (LLMs) and Multimodal Large Language Models (MLLMs) are key advancements in artificial intelligence (AI) capable of generating text, interpreting images, and understanding complex multimodal inputs, mimicking human intelligence. However, concerns arise due to their potential misuse and vulnerabilities to jailbreak attacks, where malicious inputs trick the models into generating harmful or objectionable…
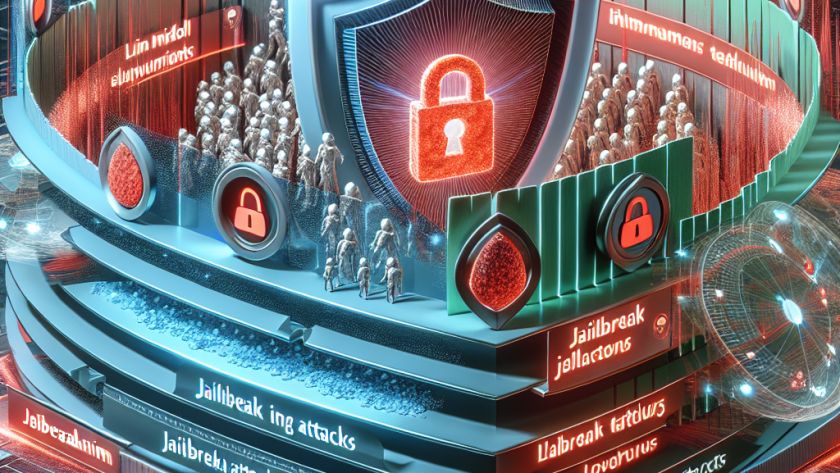