


In the domain of visual question answering (VQA), the Multi-Image Visual Question Answering (MIQA) remains a major hurdle. It entails generating pertinent and grounded responses to natural language prompts founded on a vast assortment of images. While large multimodal models (LMMs) have proven competent in single-image VQA, they falter when dealing with queries involving an…


As AI models become increasingly integrated into various sectors, understanding how they function is crucial. By interpreting the mechanisms underlying these models, we can audit them for safety and biases, potentially deepening our understanding of intelligence. Researchers from MIT's Computer Science and Artificial Intelligence Laboratory (CSAIL) have been working to automate this interpretation process, specifically…

In recent years, diffusion models have emerged as powerful assets in various fields including image and 3D object creation. Renowned for their proficiency in managing denoising assignments, these models can effectively transform random noise into the targeted data distribution. But their deployment triggers high computational costs, mainly because these deep networks are dense, which means…

Document Understanding (DU) involves the automatic interpretation and processing of various forms of data including text, tables, charts, and images found in documents. It has a critical role in extracting and using the extensive amounts of information produced annually within the vast multitude of documents. However, a significant challenge lies in understanding long-context documents spanning…

Large Language Models (LLMs) and multi-modal counterparts (MLLMs), crucial in advancing artificial general intelligence (AGI), face issues while dealing with visual mathematical problems, especially where geometric figures and spatial relationships are involved. While advances have been made through techniques for vision-language integration and text-based mathematical problem-solving, progress in the multi-modal mathematical domain has been limited.
A…

Robustness plays a significant role in implementing deep learning models in real-world use cases. Vision Transformers (ViTs), launched in the 2020s, have proven themselves to be robust and offer high-performance levels in various visual tasks, surpassing traditional Convolutional Neural Networks (CNNs). It’s been recently seen that large kernel convolutions can potentially match or overtake ViTs…

Whole-body pose estimation is an integral aspect in enhancing the capabilities of AI systems that center around human interaction. It plays a significant role in various applications such as human-computer interaction, avatar animation, and the film industry. Despite the progression of lightweight tools like MediaPipe that deliver good real-time performance, the accuracy still requires further…
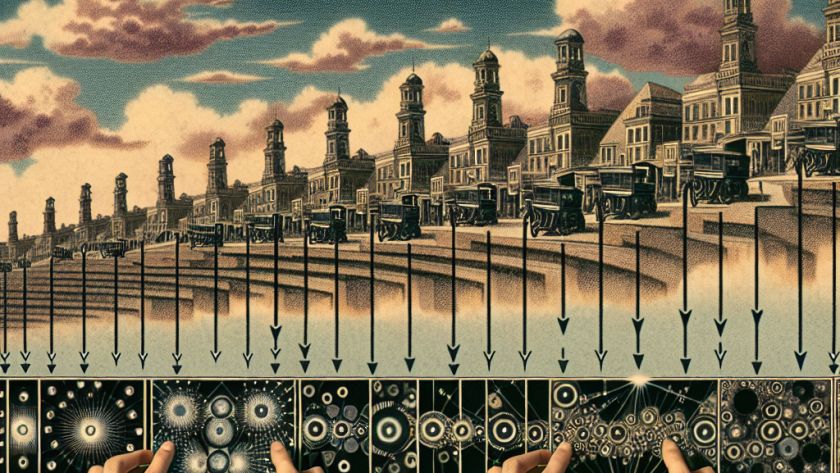
Stereo matching, a fundamental aspect of computer vision for nearly fifty years, involves the calculation of disparity maps from two corrected images. Its application is critical to multiple fields including autonomous driving, robotics and augmented reality. Existing surveys categorise end-to-end architectures into 2D and 3D based on cost-volume computation and optimisation methodologies. These surveys highlight…

Large Language Models (LLMs) have seen substantial progress, leading researchers to focus on developing Large Vision Language Models (LVLMs), which aim to unify visual and textual data processing. However, open-source LVLMs face challenges in offering versatility comparable to proprietary models like GPT-4, Gemini Pro, and Claude 3, primarily due to limited diverse training data and…

The power of Large Multimodal Models (LMMs) has shown great potential in furthering artificial general intelligence. These models are enhanced with visual abilities by harnessing vast amounts of vision-language data and aligning vision encoders. Despite this, most open-source LMMs are focused primarily on single-image scenarios, leaving complex multi-image scenarios mostly untouched. This oversight is significant…