Computer vision is a rapidly growing field that enables machines to interpret and understand visual data. This technology involves various tasks like image classification, object detection, and more, which require balancing local and global visual contexts for effective processing. Conventional models often struggle with this aspect; Convolutional Neural Networks (CNNs) manage local spatial relationships but…
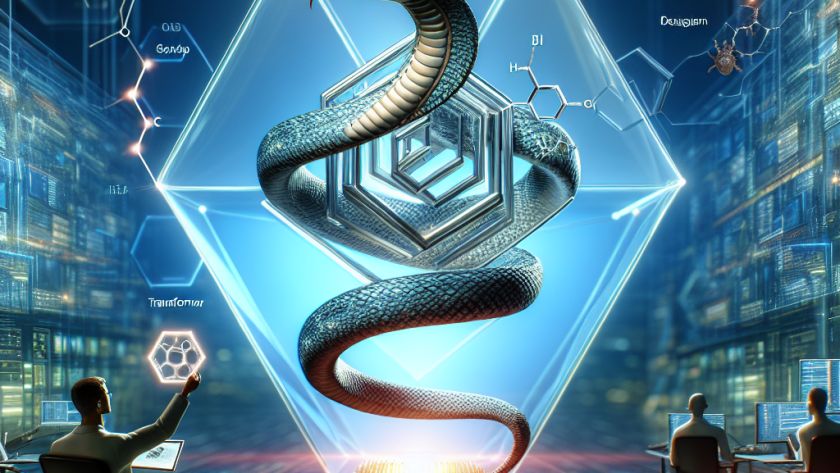