Researchers from New York University, Genentech, and CIFAR have proposed a new paradigm to address inconsistencies in supervised multi-modal learning referred to as Inter & Intra-Modality Modeling (I2M2). Multi-modal learning is a critical facet of machine learning, used in autonomous vehicles, healthcare, and robotics, among other fields, where data from different modalities is mapped to…
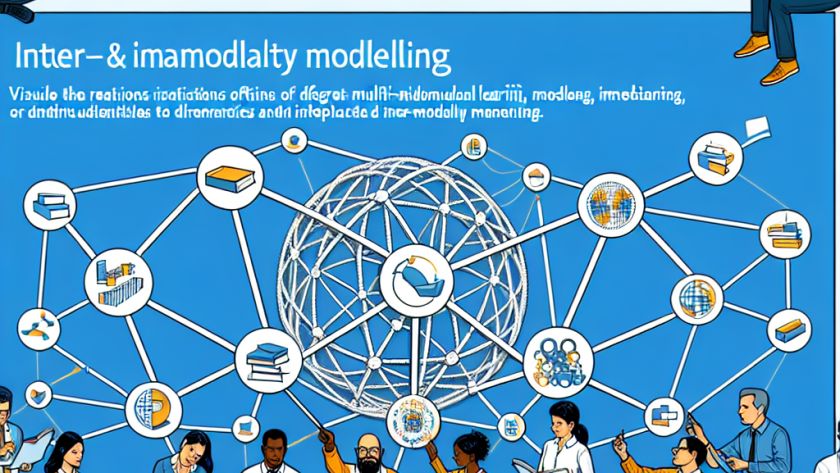