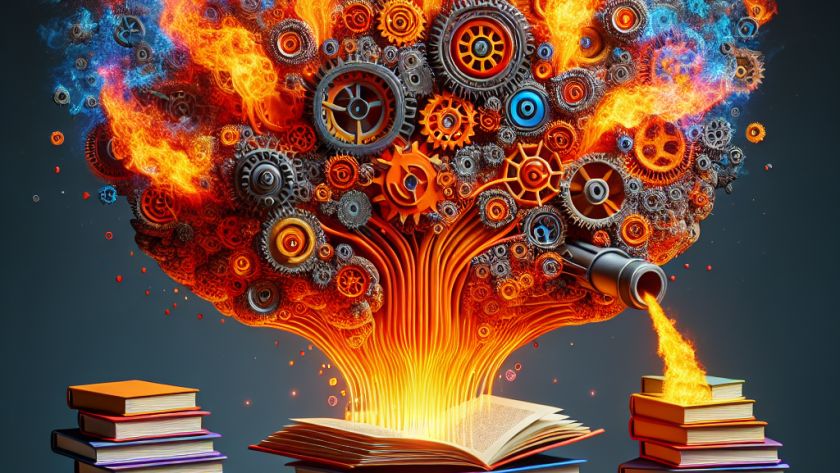
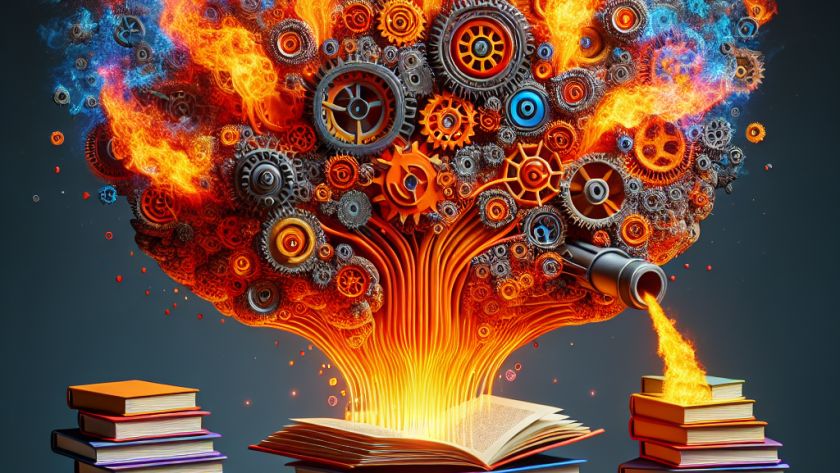




Generative models aim to replicate the patterns in the data they are trained on, often striving to replicate human actions and results. These models strive to match human proficiency in various tasks, but there is a debate over whether these models can surpass their human trainers. A new study from researchers at Harvard University, UC…

Evaluating Large Language Models (LLMs) is a difficult task, as real-world problems are quite complex and ever-changing. Conventional benchmarks often fail to provide a holistic picture of LLMs' performance. Here are some key metrics recently highlighted in a LinkedIn post:
1. MixEval: Designed to ensure balance between user queries and effective grading, MixEval combines real-world user…


Modern bioprocess development is significantly influenced by machine learning (ML), which is a part of a wide range of analytics techniques, digitalisation, and automation methods. These tools generate large sets of experimental data which are crucial in the optimisation of bioprocessing methodologies. With the help of ML, these vast datasets can be efficiently examined to…
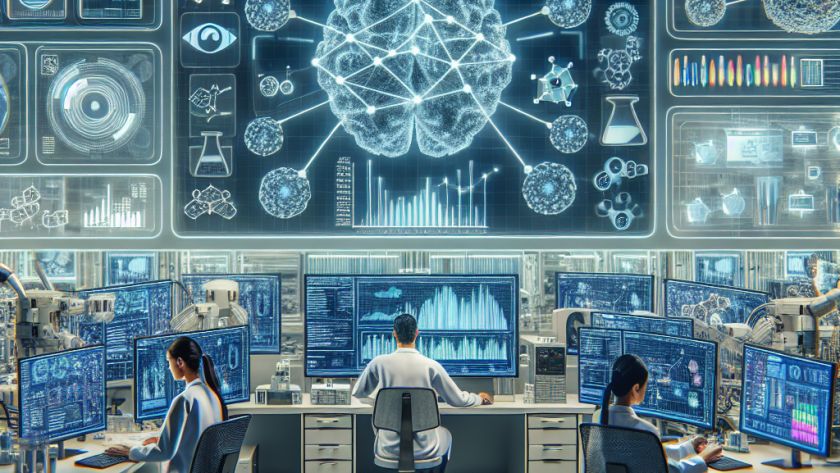
Modern bioprocess management, guided by sophisticated analytical techniques, digitalization, and automation, is generating abundant experimental data crucial for process optimization. Machine Learning (ML) techniques have proven crucial in analyzing these huge datasets, allowing for the efficient exploration of design spaces in bioprocessing. ML techniques are utilized in strain engineering, bioprocess optimization, scale-up, and real-time monitoring…

Meta's Fundamental AI Research (FAIR) team has announced several significant advances in the field of artificial intelligence, reinforcing their commitment to collaboration, openness, and responsible artificial intelligence development. With a focus on principles of excellence and scalability, the team's aim is to foster cutting-edge innovation.
Meta FAIR has launched six key research artifacts which include innovative…

Meta's Fundamental AI Research (FAIR) team has made significant advancements and contributions to AI research, models, and datasets recently that align with principles of openness, collaboration, quality, and scalability. Through these, the team aims to encourage innovation and responsible development in AI.
Meta FAIR has made six key research artifacts public, as part of an aim…

Open-source pre-training datasets play a critical role in investigating data engineering and fostering transparent and accessible modeling. Recently, there has been a move from frontier labs towards the creation of large multimodal models (LMMs) requiring sizable datasets composed of both visual and textual data. The rate at which these models advance often exceeds the availability…