Advancements in Large Language Models (LLMs) technology have burgeoned its use in clinical and medical fields, not only providing medical information, keeping track of patient records, but also holding consultations with patients. LLMs are equipped to generate long-form text compatible for responding to patient inquiries in a thorough manner, ensuring correct and instructive responses.
To…
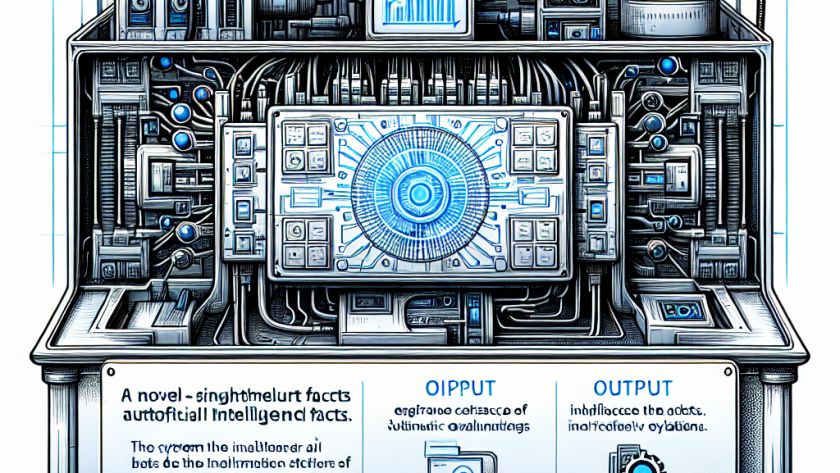