In the ever-expanding world of Federated Learning (FL), a critical challenge presents itself—optimizing hyperparameters essential for refining machine learning models. The intricate interplay of data heterogeneity, system diversity, and stringent privacy constraints introduces significant noise during hyperparameter tuning, raising questions about the efficacy of existing methods. However, a groundbreaking exploration by researchers at Carnegie Mellon…
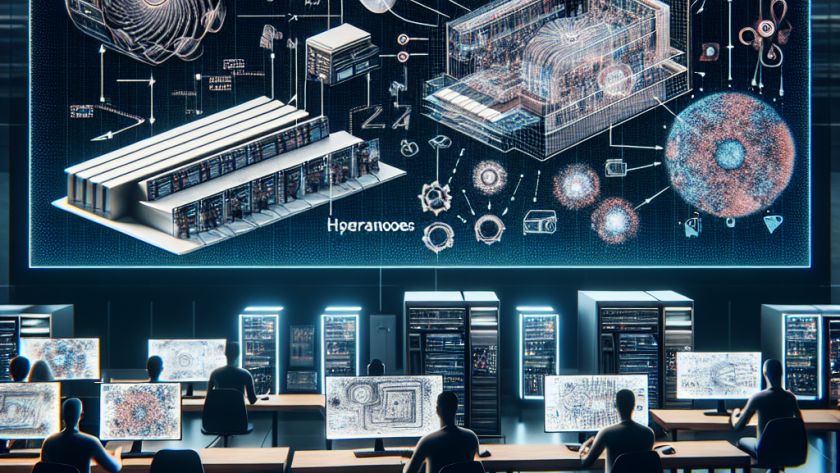