Google's AI research team, DeepMind, has unveiled Gemma 2 2B, its new, sophisticated language model. This version, supporting 2.6 billion parameters, is optimized for on-device use and is a top choice for applications demanding high performance and efficiency. It holds enhancements for handling massive text generation tasks with more precision and higher levels of efficiency…
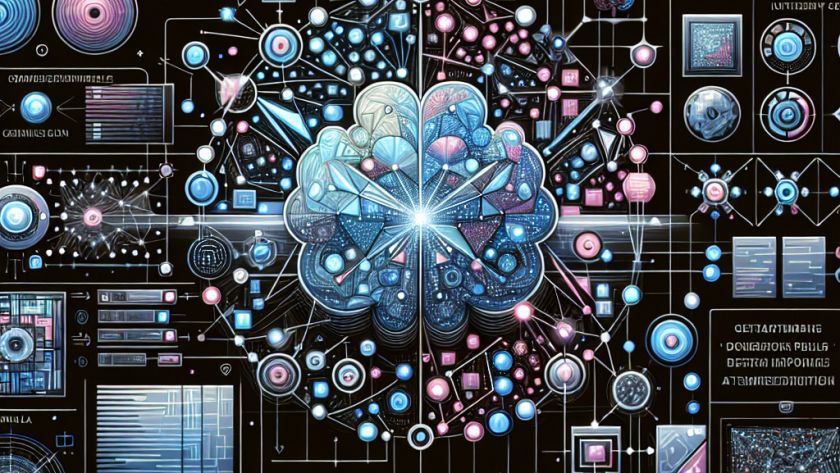