Foundation models are critical to AI's impact on the economy and society, and their transparency is imperative for accountability, understanding, and competition. Governments worldwide are launching regulations such as the US AI Foundation Model Transparency Act and the EU AI Act to promote this transparency. The Foundation Model Transparency Index (FMTI), rolled out in 2023,…
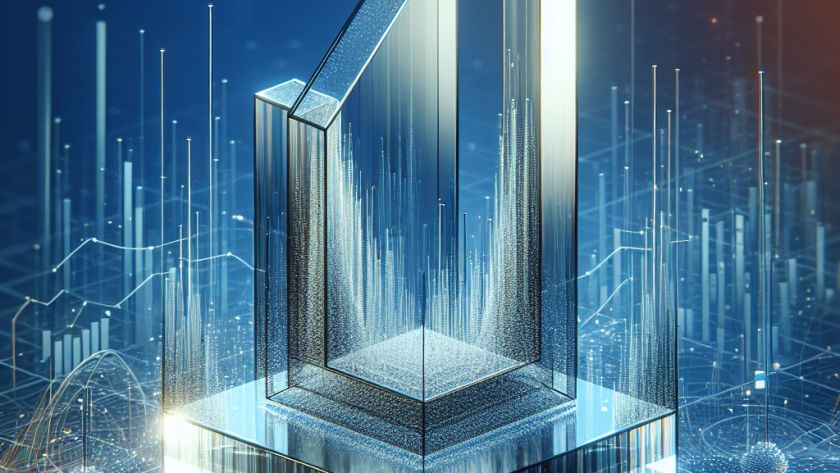