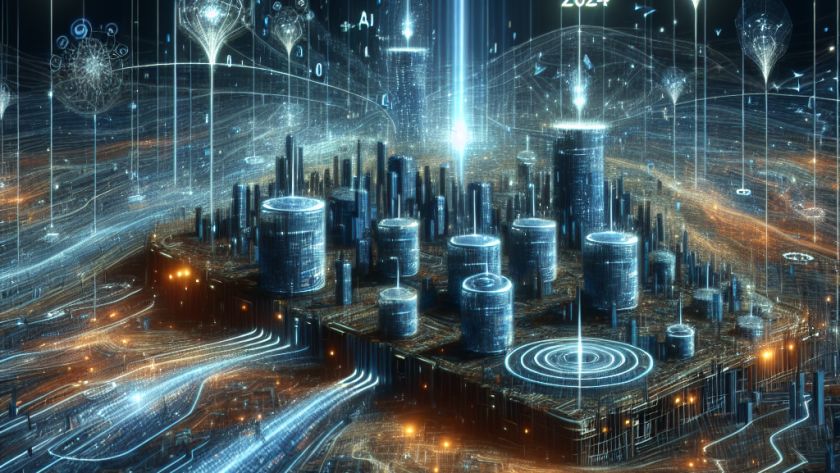
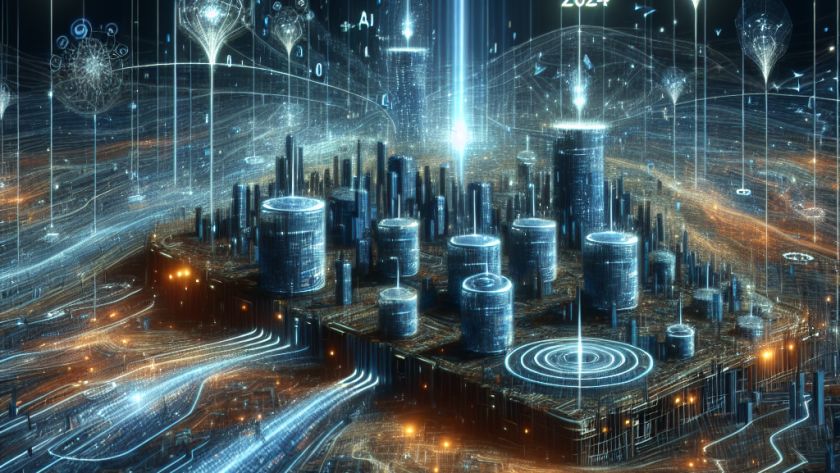


Clinical Decision Support Systems (CDSS) are data-driven digital tools that assist healthcare professionals in diagnosing diseases, prescribing treatments, and managing patient care. By harnessing data from electronic health records, CDSS offers real-time and patient-specific recommendations, ensuring patients receive optimal, evidence-based care. They range from simple notifications to comprehensive care plans, significantly enhancing decision-making processes and…

Runway has launched its newest text-to-video (T2V) generator, Gen 3 Alpha, promising improved hyperrealistic video generation in the AI-driven video production industry. The generation tool, which enables users to create expressive characters with a wide array of actions, gestures, and emotions, marks an upgrade from the company's previous Gen 2 T2V tool, offering advanced features…



DuckDB is a high-performance in-process SQL database management system (DBMS). It is designed for complex and resource-intensive data analysis tasks, with a focus on speed, reliability, and user-friendliness. Its SQL dialect goes beyond basic SQL functionality, supporting complex queries such as nested and correlated subqueries, window functions, and unique data types like arrays and structures.
One…
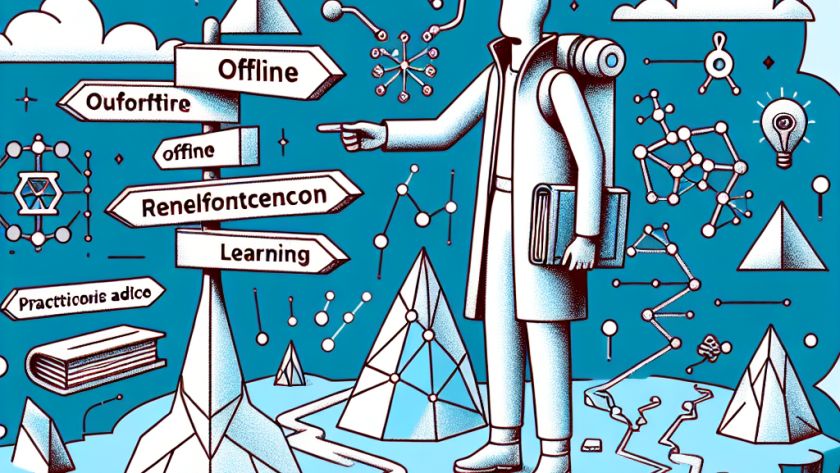

Data-driven techniques, such as imitation and offline reinforcement learning (RL), that convert offline datasets into policies are seen as solutions to control problems across many fields. However, recent research has suggested that merely increasing expert data and finetuning imitation learning can often surpass offline RL, even if RL has access to abundant data. This finding…

Researchers from New York University, Genentech, and CIFAR are pioneering a new approach to multi-modal learning in an attempt to improve its efficacy. Multi-modal learning involves using data from various sources to inform a target label, placing boundaries between the sources to allow for differentiation. This type of learning is commonly used in fields like…
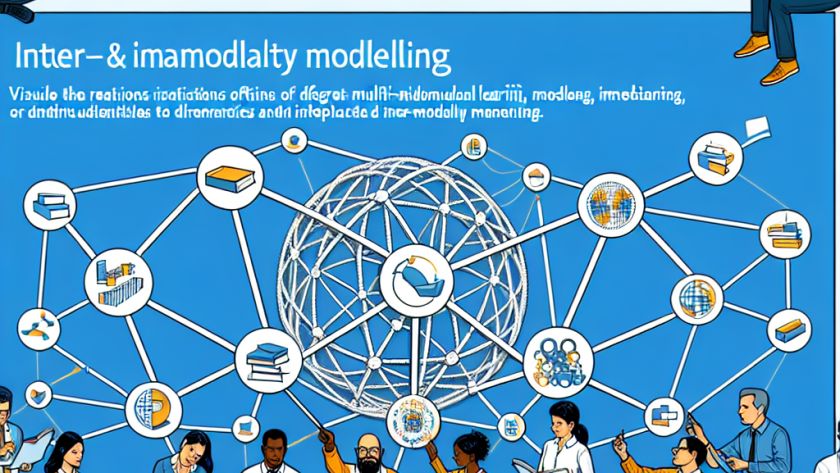
Researchers from New York University, Genentech, and CIFAR have proposed a new paradigm to address inconsistencies in supervised multi-modal learning referred to as Inter & Intra-Modality Modeling (I2M2). Multi-modal learning is a critical facet of machine learning, used in autonomous vehicles, healthcare, and robotics, among other fields, where data from different modalities is mapped to…